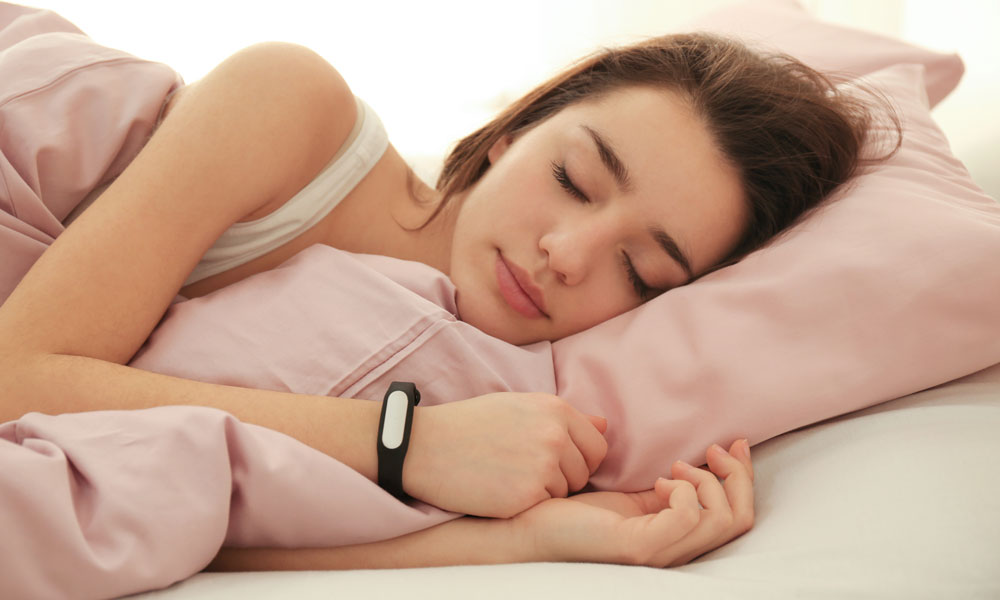
Relieving sleep labs with AI
The classification of sleep stages is an important basis for the care of people with sleep disorders. Researchers from Salzburg Research and the University of Salzburg have analysed different machine learning approaches for sleep stage classification based on interval and respiration data. The data for the developed models can be collected with low-cost sensor systems. This makes data collection at home possible to relieve clinical sleep labs.
Sleep plays a crucial role for our organism and is essential for our physical and mental well-being. Non-restorative sleep is associated with impairments in social, occupational or other areas of functioning, leading to massive socio-economic burdens. Alarmingly, general sleep disorders are widespread and affect about one third of the adult population.
Relieving the burden on sleep labs through automated sleep assessment
However, the prevalence of sleep disorders is matched by a significant shortage of qualified sleep laboratories, which often leads to delays in diagnosis. In sleep labs, sleep is measured based on polysomnography (PSG) and classified into different sleep stages by experts – a personnel-intensive, time-consuming and expensive process.
New, low-cost measurement technologies and automated sleep assessment routines can help to address the widespread sleep problems in modern societies. Researchers from Salzburg Research and the University of Salzburg have now analysed the generalisability of machine learning approaches to classify sleep stages.
Sleep data that can be obtained at home
During sleep, basic physiological signals such as heart rate variability (HRV) and respiratory rate change so that sleep can be reliably classified into three to four sleep stages based on these signals alone. All features used for automated sleep stage classification are calculated from such interbeat intervals (IBI) or respiration data. “In the future, it should be possible to collect these data with inexpensive sensor systems, so that the data for sleep stage classification can be collected directly from the test persons at home and not in clinical facilities,” says Salzburg Research researcher Stefan Kranzinger. “Overall, the results show that we can use machine learning to make good statements about sleep stages. We are well on the way to being able to use automated technologies to supplement the care of people in sleep labs in the future. Further research will lead to a further refinement of the classifications.”
More Information:
- Press release (in German): Entlastung von Schlaflaboren: Schlafstadien zuhause mit KI klassifizieren
- Forschungsprojekt: Virtual Sleep Lab – Virtuelles Schlaflabor
- Publication: Stefan Kranzinger, Sebastian Baron, Christina Kranzinger, Dominik Heib, Christian Borgelt (2023): Generalisability of sleep stage classification based on interbeat intervals: validating three machine learning approaches on self-recorded test data. In: Behaviormetrika (2023).