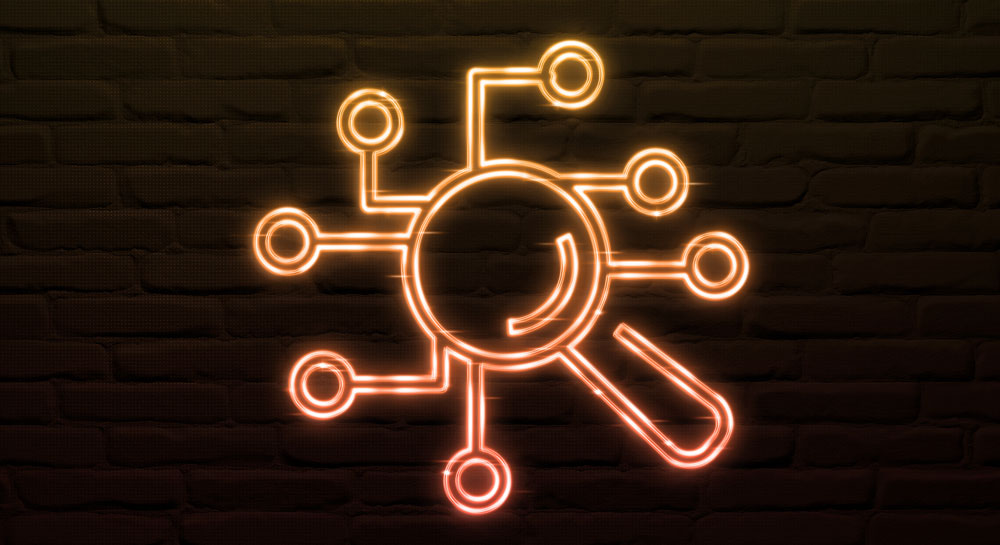
Detecting anomalies in real-time communication networks
The timely detection of anomalies in communication networks is especially important for time-critical applications. The more time it takes to detect and react to an anomaly, the more serious the consequences can be. Salzburg Research has developed a software architecture that can detect and react to anomalies in real time using machine learning.
Real-time communication networks are becoming increasingly important, especially in cyber-physical systems in critical areas such as state-of-the-art production facilities or smart energy grids. Analogously, the use of machine learning will play an increasing role in order to be able to detect anomalies in time.
“The detection of anomalies is an example of the successful application of machine learning methods. Algorithms independently recognise patterns and regularities in data sets and can develop solutions from them,” says Peter Dorfinger, head of the Intelligent Connectivity research group at Salzburg Research Forschungsgesellschaft.
Machine learning meets real-time networks
Salzburg Research has developed an end-to-end real-time architecture for anomaly detection. In this architecture, the collection and transmission of the required data, the analysis of this data in a machine learning model and the subsequent reaction are carried out in a defined time. While previous approaches already use machine learning for anomaly detection, the researchers at Salzburg Research Forschungsgesellschaft also bring their expertise in real-time communication networks to bear in their approach.
So-called “Autoencoder Neuronal Networks” (ANNs), a special type of artificial neural network, are used. They can process unstructured data. “The learning process of the neural network is unsupervised. This means that no labelling of the input data is required. This is a big advantage, as this pre-processing of data is usually very time-consuming,” Dorfinger continues.
Proof-of-Concept: Neural network causes reconfiguration
The proposed solution from Salzburg Research was designed for two use cases: Firstly, for the detection of anomalies in the network data with real-time reconfiguration of the real-time Ethernet network. And secondly, for detecting anomalies in machine data with real-time reconfiguration of industrial machines. A proof-of-concept was implemented in the lab. “After our ANN detects an anomaly, it triggers a reconfiguration of the network flows, such as switching off a network path, switching to another network path, or reconfiguring the machines, for example using new parameter settings,” says Dorfinger.
In the future, measurements will be taken to evaluate the proposed architecture in terms of actual response time from anomaly detection to reconfiguration of the network or machine. The results will then be compared with measurements in existing anomaly detection systems.
To the publication: Christian Maier, Jia Lei Du, Stefan Fahrthofer, Peter Dorfinger (2021): An Architecture for Detection of Anomalies in Deterministic Time within Real-Time Communication Networks. In: CORETA 2021: Advances on Core Technologies and Applications.