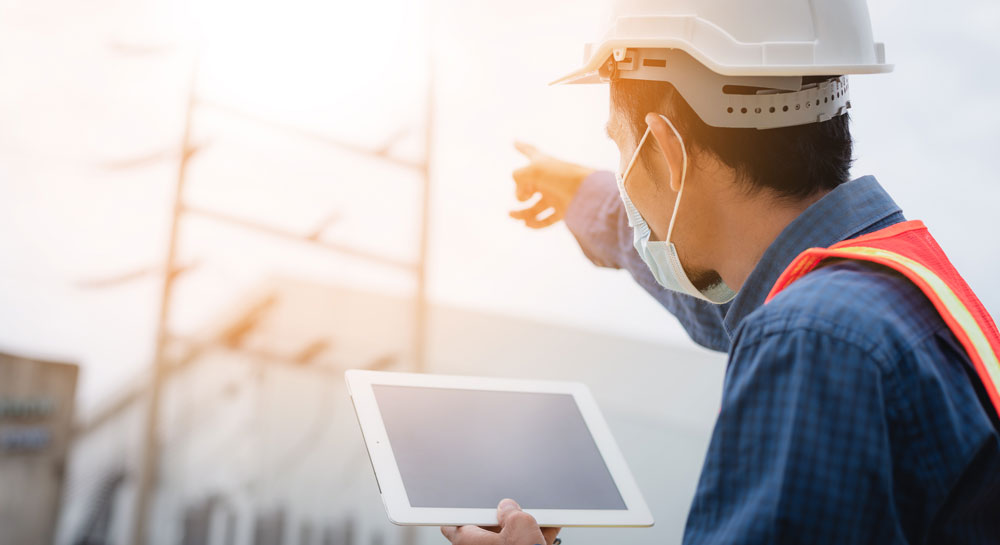
Automated fault diagnostics in the power grid
Salzburg Research tapped the potential of telecom diagnostics data for automated fault diagnostics in the low-voltage network for an electricity distribution network operator: with the help of machine learning, the cause of approximately 70 per cent of low-voltage network faults can be detected automatically without additional hardware.
The starting position
The client operates approx. 5,300 transformer stations throughout the supply area. The sooner a fault can be detected, the quicker the fault repair can be initiated. Therefore, the electricity distribution network operator wanted to extend its fault diagnostics in the high and medium voltage network to the low voltage network. Until now, customers had to report faults by telephone.
In the low-voltage networks of every transformer station there are also telecom devices that can be used for fault diagnosis. However, it was unclear whether the available telecom network diagnostic data contained sufficient information to detect a fault in the low-voltage network.
Machine learning helps identify faults
Salzburg Research answered these questions for the distribution network operator using machine learning. Based on the information of the existing telecom fault, it is automatically identified whether it is a telecom fault or a fault in the distribution grid. The analysis showed that automatic detection based on telecom diagnostic data is possible for approximately 70 percent of low-voltage grid faults.
By making telecom diagnostic data usable, low-voltage grid faults can thus be detected live without additional investment in hardware. As a result, troubleshooting can be started earlier and the time needed to interrupt the supply can be reduced.
More information: